Key Deep Learning Models for iOS Apps Natural Language Processing (NLP) Models NLP models enable apps to understand and generate human-like text, supporting features like chatbots, sentiment analysis, and real-time translation. Top NLP Models for iOS: • Transformers (e.g., GPT, BERT, T5): Powerful for text generation, summarization, and answering queries. • Llama: A lightweight, open-source alternative to GPT, ideal for mobile apps due to its resource efficiency. Example Use Cases: • Building chatbots with real-time conversational capabilities. • Developing sentiment analysis tools for analyzing customer feedback. • Designing language translation apps for global users. Integration Tools: • Hugging Face: Access pre-trained models like GPT, BERT, and Llama for immediate integration. • PyTorch: Fine-tune models and convert them to Core ML for iOS deployment. Generative AI Models Generative AI models create unique content, including text, images, and audio, making them crucial for creative apps. Top Generative AI Models: • GANs (Generative Adversarial Networks): Generate photorealistic images, videos, and audio. • Llama with Multimodal Extensions: Handles both text and images efficiently, ideal for creative applications. • VAEs (Variational Autoencoders): Useful for reconstructing data and personalization. Example Use Cases: • Apps for generating digital art and music. • Tools for personalized content creation, like avatars or wallpapers. • Text-to-image applications for creative projects. Integration Tools: • RunwayML and DeepAI APIs for pre-trained models. • Core ML for on-device deployment of generative tasks. Voice-Changing with Deep Learning Voice-changing technologies powered by deep learning enhance entertainment, gaming, and content creation. Top Voice Models for iOS: • WaveNet: Produces realistic voice transformations and high-quality audio. • MelGAN: Lightweight and efficient, ideal for real-time applications. • Voice Conversion Models (VCMs): Transform one voice to mimic another while preserving speech content. Example Use Cases: • Gaming apps offering immersive voice transformations. • Content creation tools for podcasting or video editing. • Accessibility apps for personalized voice adjustments. Practical Implementation: 1. Model Selection: Choose lightweight models like MelGAN for on-device processing. 2. Optimization: Quantize and prune models to reduce size for mobile deployment. 3. Integration: Use Core Audio or AVFoundation for seamless audio processing and Core ML for AI workflows. 4. Diffusion Models for Realistic Content Creation Diffusion models are widely used for generating realistic images, making them a powerful tool for creative apps. Challenges of Diffusion Models: • Large sizes (often hundreds of megabytes or more) can make them difficult to deploy directly on mobile devices. How to Optimize Diffusion Models for iOS: 1. Model Quantization: Reduce size without significantly impacting quality using tools like PyTorch’s quantization toolkit. 2. Cloud-Based Inference: Offload heavy computation to cloud services like AWS or Google Cloud AI, delivering results to the app in real time. 3. Core ML Conversion: Use coremltools to convert and optimize diffusion models for iOS, leveraging Apple’s Neural Engine for improved performance. 4. Hybrid Deployment: Split the model—keep lightweight preprocessing on-device and offload heavy processes to the cloud. Use Cases: • AI-powered design tools for creating images or videos. • Apps for personalized content generation (e.g., user-specific art). Advantages of Using APIs in iOS Development…
Thank you for reading this post, don't forget to subscribe!Deep Learning Models integration for iOS Apps – briefly explained – Day 52
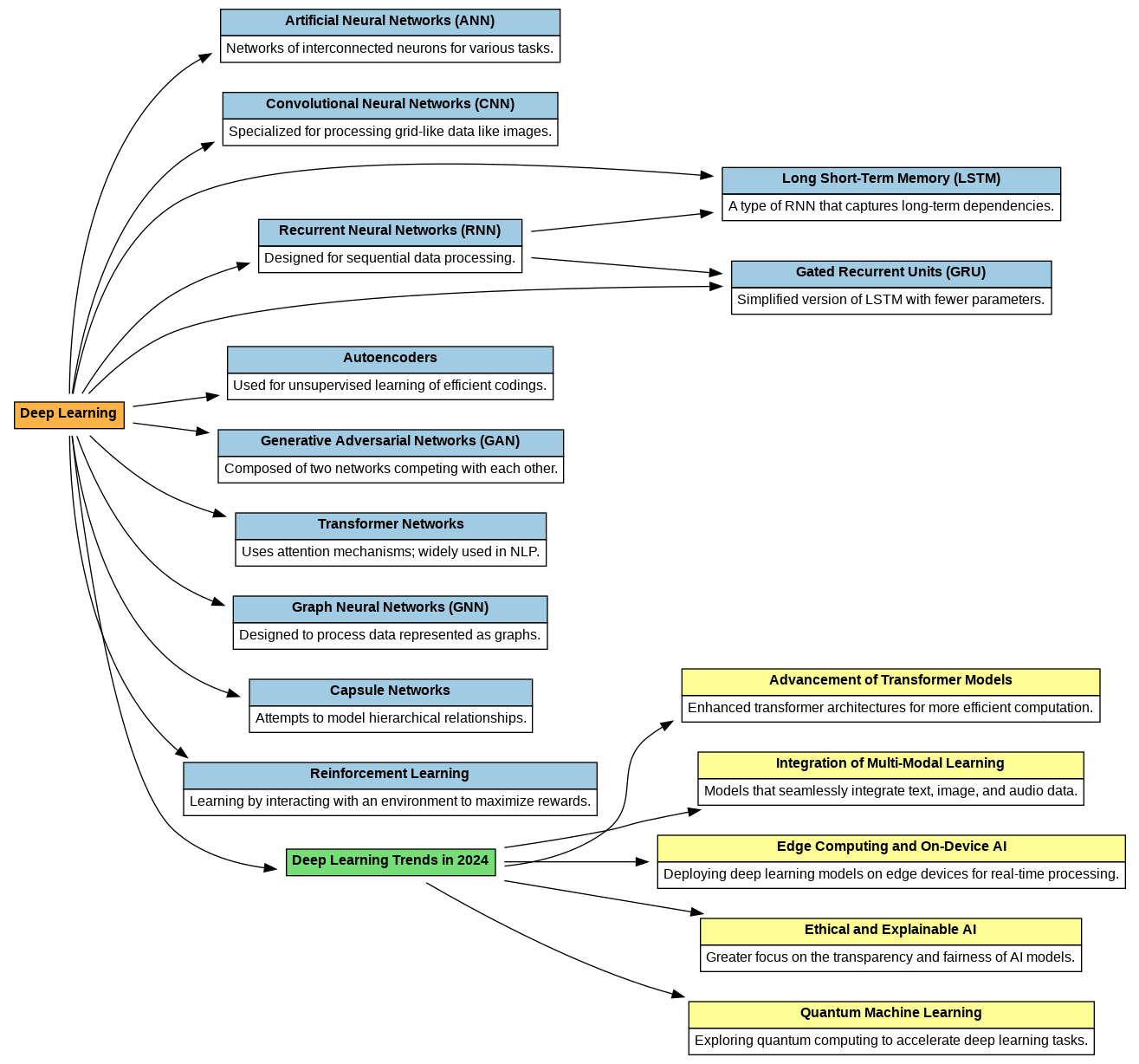